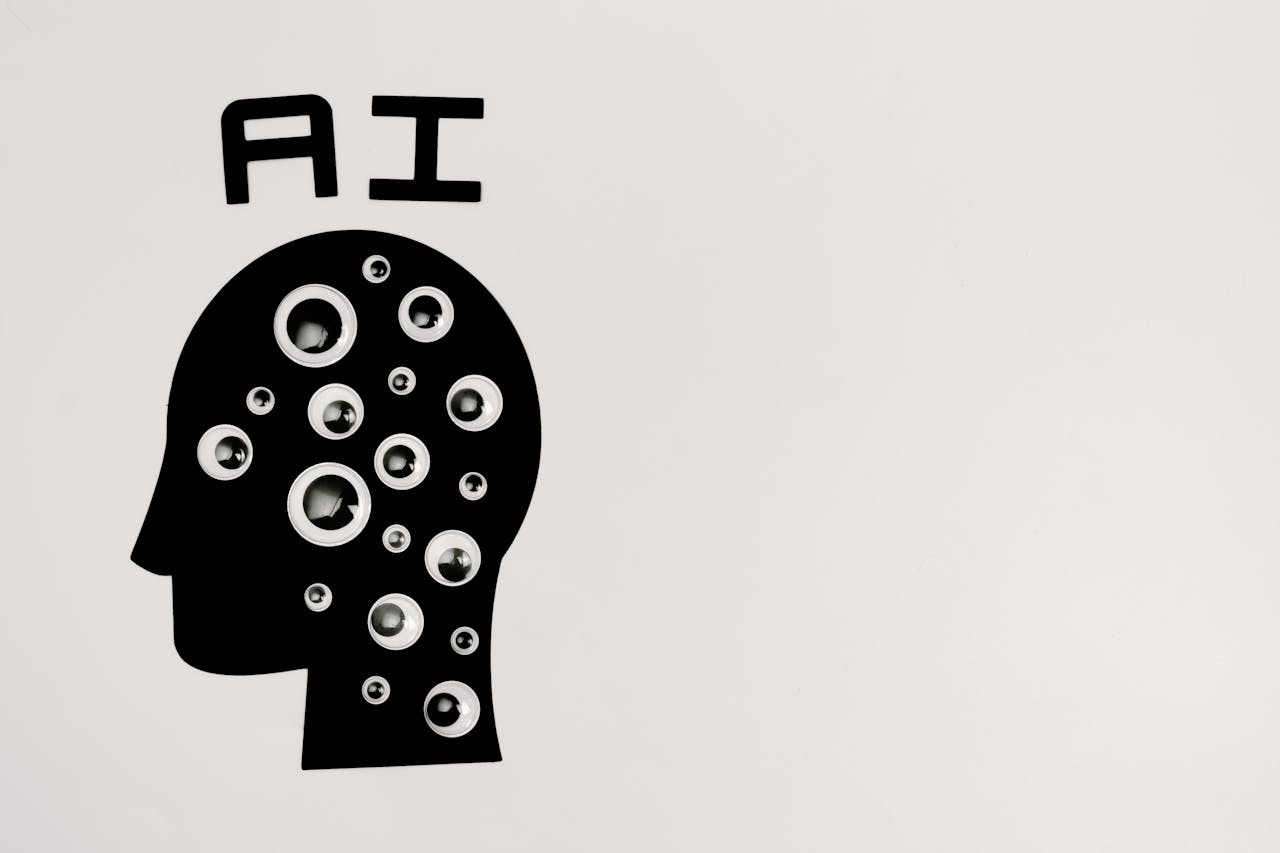
The debate over the future of large language models (LLMs) — like ChatGPT — is heating up, with both skeptics and enthusiasts taking firm stands. But amidst the clash of opinions, there’s a significant dose of overconfidence on both sides.
Former OpenAI employee Leopold Aschenbrenner recently stirred the pot by suggesting that we might be just a few years away from LLM-based general intelligence. This scenario, he argues, could revolutionize remote work, urging a push to develop it before China takes the lead.
Aschenbrenner’s analysis reflects a common belief that as LLMs grow larger and more sophisticated, their errors will diminish, paving the way for artificial general intelligence. This perspective, dubbed “scale is all you need,” hinges on the idea that more data and computing power will solve the limitations of current models.
However, prominent skeptics like Yann LeCun and Gary Marcus remain unconvinced. They point out persistent flaws in LLMs, such as their struggles with logical reasoning and tendency towards “hallucinations,” suggesting that scaling up may not provide the breakthroughs we anticipate.
The truth likely lies somewhere in between. While scaling has undoubtedly improved LLM performance across various tasks, predicting the trajectory of AI development is complex and fraught with uncertainty.
Moreover, the rush to predict AGI’s arrival overlooks the fundamental differences between LLMs and human intelligence. Comparing them on a linear graph, as Aschenbrenner does, oversimplifies the challenges ahead.
AI safety advocate Eliezer Yudkowsky aptly notes our ignorance regarding the distance to human-level research on this graph. With so much unknown, making confident predictions about AGI’s timeline is premature.
Navigating this uncertainty requires humility and open-mindedness. Rather than doubling down on bold predictions, we should embrace a range of possibilities and focus on understanding and mitigating potential risks.
Whether AGI arrives in 2027 or beyond, the implications demand serious consideration. Aschenbrenner’s scenario, while speculative, highlights the need for robust policy responses to emerging AI capabilities.
In this evolving landscape, acknowledging our limitations is the first step towards better preparation and response. While the allure of sensationalist timelines may capture attention, it’s the underlying concerns about our readiness that merit serious attention.
In the end, our ability to grapple with the challenges of AI hinges on our willingness to confront uncertainty and adapt to new information. Only then can we navigate the complex terrain of AI development with clarity and purpose.